Good demand forecasts are the basis for comprehensive supply chain planning and the resulting optimization of processes. The more accurate the forecasts, the lower the safety stocks, depreciation and tied capital.
With forecasting procedures based on machine learning (ML), an artificial system learns to recognize patterns in existing company data, to assess future data, to make decisions and to warn of errors. But what is really important and how does an introduction to ML-based demand planning work? We have summarized the ten Do’s for you.
1. Check and Optimize Your Data Situation!
For an algorithm to draw valid conclusions from data – i.e. to lay the foundation for machine learning – a critical amount of data must be available in the right quality. Have a potential assessment carried out to clarify how well your company is positioned in this respect.
2. Develop a Comprehensive Understanding of Data!
Only those who know and understand the content of their data can provide meaningful and complete data sets to the ML algorithm – a central prerequisite for the success of ML-based procedures. An algorithm can only be as good as the database from which it learns.
3. Build Up Your ML-Experts!
As with conventional statistical methods of demand planning, the calibration of ML methods requires methodological know-how and is a continuous optimization process. You should therefore build up appropriate experts in your team who are familiar with the methods and can continuously adjust the set screws.
4. Consider the Individual Requirements!
There is no general-purpose method. Rather, an ML algorithm must be selected sensibly based on the application case. General conditions to be considered here are, for example, the demand pattern, the required forecast horizon, the available data basis and the accepted calculation effort.
5. Take into Account the Effort of Data Preparation!
In order to be able to train ML approaches, the data must be prepared in relation to the application case with a high expenditure of time. Experience shows that significantly more than half of the total effort is spent on data collection, data cleansing and structuring, the creation of training data sets, etc. Therefore, do not underestimate the time and effort required to create a good database.
6. Bring in Domain Knowledge!
In order to successfully implement an ML-based forecast in the application, technical and methodological specialists must be in close contact. Form a forecast team to bring together the knowledge of your sales and ML experts. This is the only way to create sufficient acceptance among your employees and to teach the algorithm meaningful decisions.
7. Keep Your ML-Team Up to Date!
To improve the performance of ML algorithms, intensive feature engineering is required. The data is prepared in such a way that the algorithm can detect possible correlations between an influencing variable (e.g. marketing campaign) and the result variable (e.g. sales volume) in the first place. Therefore, make sure that new developments from the daily business reach your ML team!
8. Train!
The longer a model is trained, the more accurately it can take into account the characteristics of the data set and the more accurate your forecast will be.
9. Use the New Possibilities!
Especially for the demand forecast there are now various ML-based procedures and empirical values. Exchange experiences with other companies about their experiences in this area and involve external experts.
10. ML is not an End in Itself: Use the Best Procedure!
Companies, customers and products are different. Therefore, it is always necessary to analyze which methods can achieve the best forecast quality. Sometimes conventional statistical methods deliver better results than the latest ML algorithms! So only use an ML-based demand forecast if you see a clear added value in using these methods.
Knowing today which demand is realistic tomorrow, in a month or in a year? Which company does not want that? But don’t forget that forecast models are merely possible scenarios that occur with a certain probability – but if they are based on meaningful data, you can rely on a high quality of decision-making. You may even be able to take into account the probabilities of future pandemics. In this way you would be prepared for Corona 2.0 in case of an emergency.
Our Experts
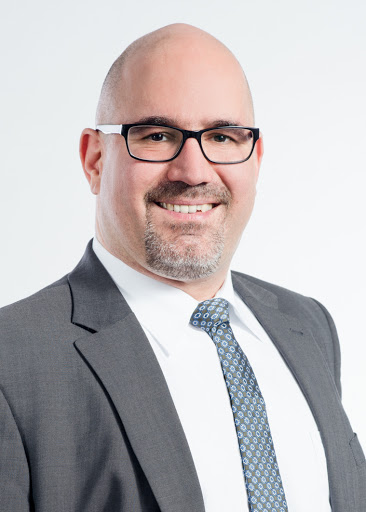
Fraunhofer Institute for Material Flow and Logistics
and his colleagues Saskia Wagner Sardesai, Josef Kamphues, Philipp Klink, Nikolas Moroff