In today’s fast-paced and competitive business landscape, companies are constantly seeking tools and strategies to gain a competitive edge and make informed decisions. One such tool that has gained significant importance is logistic growth modeling. Logistic growth models offer a valuable framework for understanding growth patterns, predicting future trends, and making strategic business decisions. This article explores the history, interdisciplinary applications, and strategic importance of logistic growth models in businesses.
Understanding the Past to Predict the Future
Logistic growth models, also known as sigmoidal growth models or S-curves, have their roots in ecology. The concept of logistic growth was first introduced by Belgian mathematician Pierre-François Verhulst in the mid-19th century as an adaptation of the Malthusian (exponential) growth model, which is unrealistic due to limited niche capacity or costs of complexity in many scenarios. Verhulst proposed that population growth tends to follow an S-shaped curve, characterized by an approximately exponential initial rapid growth phase, followed by a gradual slowdown until it reaches a stable carrying capacity.
Beyond Population Dynamics
While logistic growth models originated in the field of population biology, their applications extend far beyond. These models have found interdisciplinary applications in diverse fields such as economics, marketing, technology adoption, and innovation management. By capturing the growth dynamics of various phenomena, logistic growth models offer insights into market trends, product adoption patterns, and the life cycles of products and services.
In the realm of business, logistic growth models play a crucial role in identifying mid- to long-term growth trends. By analyzing historical data and fitting it into a logistic growth model, businesses can forecast future growth patterns, estimate market saturation points, and predict demand for products or services. This information is invaluable for strategic decision-making, allowing companies to allocate resources effectively, plan expansion strategies, and optimize their product portfolios.
Similar to technological trends, the life cycle of a product or service can be described using a logistic growth curve. Initially, products experience a slow adoption phase, followed by rapid growth, and eventually, the growth rate levels off as the market matures. By applying logistic growth modeling to the product life cycle, businesses can identify the stage at which a product or service is currently positioned, enabling them to make informed decisions regarding product development, marketing strategies, and resource allocation.
Empowering Strategic Business Decisions
Logistic growth models offer a powerful tool for businesses to understand growth dynamics, forecast future trends, assess the life cycles of existing products, and make informed strategic decisions. By utilizing these models, companies can identify market saturation points, gauge the potential for new products or services, and optimize their business strategies accordingly. Logistic growth modeling serves as a compass for innovation management, enabling businesses to stay ahead of the curve and adapt to changing market conditions. As businesses navigate the complex landscape of growth and competition, logistic growth models emerge as a vital tool for unlocking their full potential and achieving sustainable success.
Incorporating logistic growth modeling into business strategies empowers companies to assess the risk of disruption, seize opportunities, and chart a path towards long-term growth and prosperity. With the ability to understand growth patterns and anticipate market trends, businesses can stay agile, make data-driven decisions, and pave the way for innovation and success in the dynamic business world.
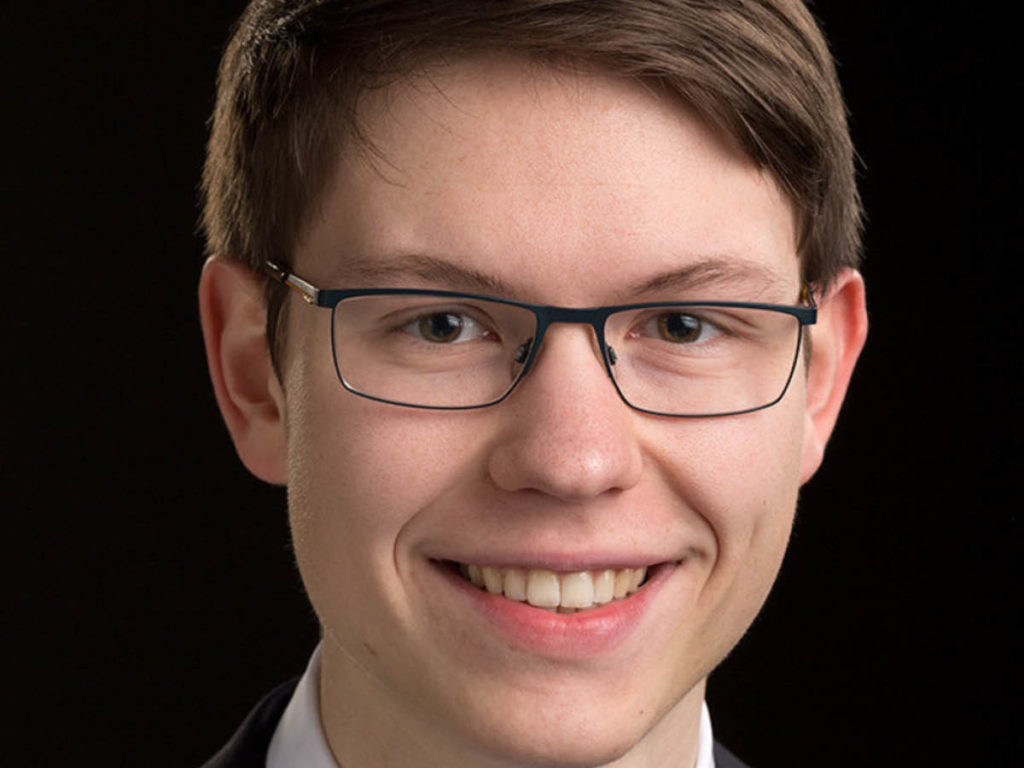
Louis Steinmeister
is a scholarship holder at the Graduate School of Logistics and is doing his doctorate in cooperation with Infineon Technologies AG.